Motion detection is the magic ingredient in the current wave of do-it-yourself home security cameras. Thanks to built-in sensors that vigilantly monitor their field of view for movement, you can go about your daily business confident that you’ll be automatically alerted to any suspicious activity in your home. Without it, you’d have to keep your eyes glued to your camera’s feed on your phone or computer—hardly a practical security solution.
But motion detection can be a puzzling and frustrating feature. Too many alerts is annoying (and a waste of time), while too few leave you wondering if the camera is working at all. Some motion sensors get tripped by events that aren’t even motion, such as a change in the lighting. And how do you keep Fido and Fluffy from setting the darn thing off all the time?
To help you better understand and address these issues, we’ve put together this primer on motion detection technology.
Two types of detection
Smart devices can employ any number of technologies—light pulses, thermal measurements, and radio waves, to name just a few—to detect motion. But consumer security cameras most commonly use one of the following two methods:
Passive Infrared (PIR):
Passive infrared sensors monitor for the ambient heat emitted by all living beings. That’s why it’s sometimes referred to as “heat vision.” When a PIR sensor detects sufficient movement of these warm “objects” and/or a significant temperature differential between the object and the background scene, it signals the camera to start recording and send an alert to the user.
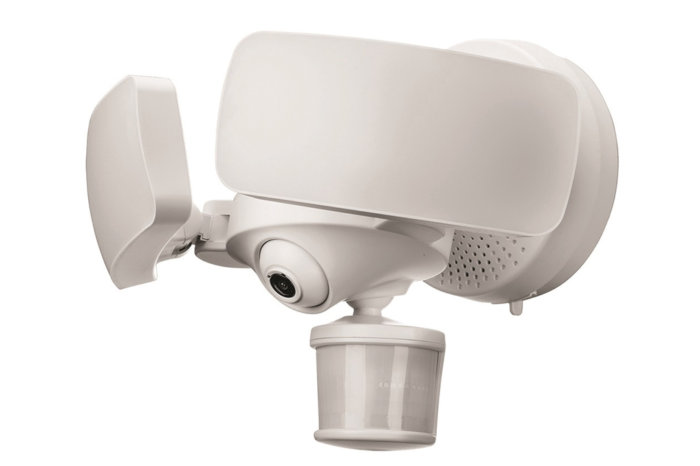
The Maximus Camera Floodlight is equipped with a passive infrared motion sensor (the semicircular object hung below the camera).
Computer vision (CV):
Computer vision is a broader approach wherein a camera’s software analyzes sequential frames of live video for differences and registers a motion event when a big enough change is detected.
The CV approach includes many different methods that depend on the desired outcome and technical preference of the team that designs it, says Andrey Katsman, Sr. Director of Software Engineering at Canary. One of the easiest, he says, is to “look for a significant change in pixels over short periods of time, compared to the longer term average to gain a crude ‘something happened’ signal.” Another way is to track groups of pixels and try to identify directional patterns—there was a blue dot here one frame ago, but now there is a blue dot one pixel to the left, for example—in an effort to recognize moving objects.
But the idea behind all CV approaches is the same: detect motion and, if possible, determine the shape of the object that moved. More advanced methods can then be added to classify the moving object—it was a person, an animal, a vehicle, or a tree—in order to determine which detection signals are important to the user and should be highlighted (the person or vehicle, for instance) and which are irrelevant and should be suppressed (animals and trees).
Pros and cons
Each of these motion detection methods has its advantages and disadvantages.
Because PIR sensors monitor for body heat, they tend to more reliably filter out inconsequential activity, such as curtains fluttering in the breeze from a nearby fan or a change in the light streaming through a window, than CV-based cameras.
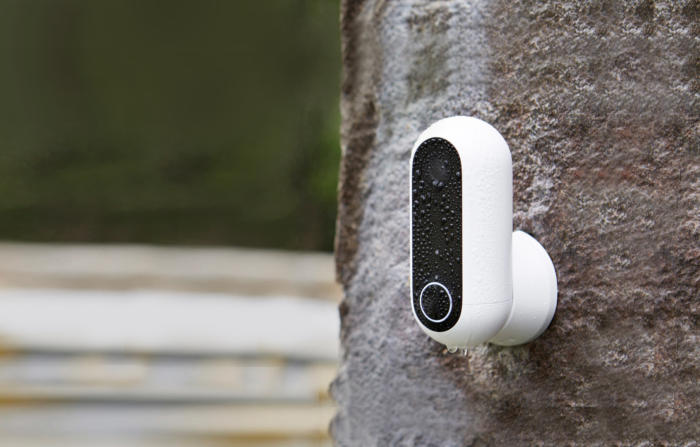
The Canary Flex home security camera uses a PIR motion sensor to enable it to run on battery power.
PIR is also very power efficient, so it’s often used in battery-powered cameras, keeping them in a low-power non-recording state until the PIR sensor detects motion. At that point, they switch to a high-power recording state for a fixed period of time—to capture a 30-second video clip, for instance—or until motion stops.
But because PIR-based cameras spend the bulk to their time low-power mode, they can take more time to wake up and start recording than CV-based cameras. That means you may miss part of the action that triggered the motion alert, such as an intruder’s approach when their face is most visible. That matters when you’re talking about forensic evidence. They are also, by nature of the technology, incapable of detecting motion through glass so they’re not suitable for scenarios such as monitoring your yard with an indoor camera aimed through a window.
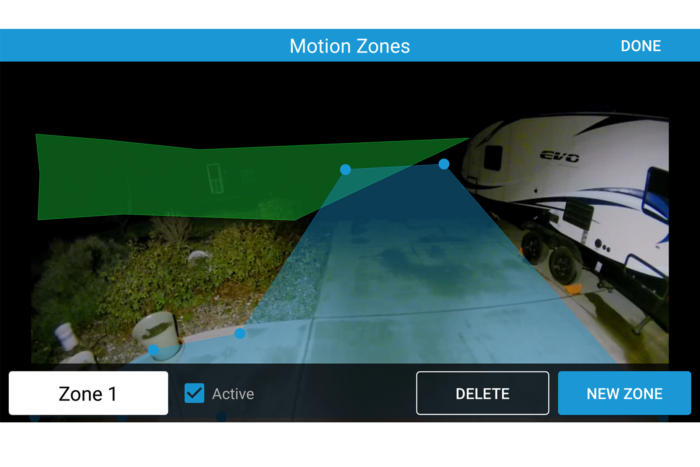
The Ring Floodlight Cam, shown at the top of this story, is supremely flexible when it comes to defining motion detection zones.
The main advantage of CV motion detection is it allows for greater analysis of the scene, and, as stated earlier, for identification of the object creating the motion through advanced features like person detection or facial recognition (more on these in a moment). But many of the algorithms that make this possible are too computationally intensive to run locally on the camera. Instead, they’re computed on remote servers in the cloud, where they often can only be unlocked only with a paid subscription. That can add significantly to the total cost of ownership of the camera.
CV detection is also dependent on the “quality” of the software algorithms, says Guardzilla Chief Revenue Officer Terry Bader. This renders CV-detection more vulnerable to false alarms, since any large change—a spinning ceiling fan, leaves blowing outside a window, a change in ambient lighting—can register as motion.
Fixing false alerts
Indeed, false motion detection alerts are probably the biggest sore point for home security camera owners. Receiving an onslaught of notifications on your phone, only to find out your camera’s sensor was tripped not by a burglar but a light snowfall or a pet wandering into its field of view, gets tedious quickly and pushes many users to deactivate their device’s most important feature.
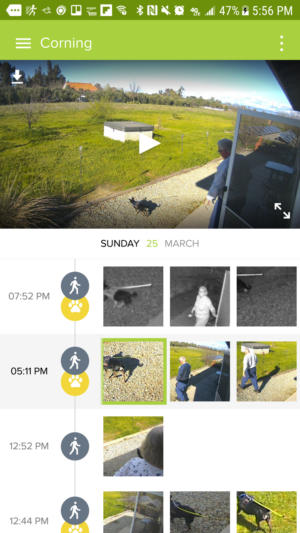
The Netatmo Presence home security camera can determine if the motion it detects is caused by people, animals, or even cars.
Camera manufacturers offer a number of ways of reducing the incidence of false alarms, each with varying degrees of success. Camera’s using PIR motion detection usually allow you to adjust the sensitivity level within the companion app, so that more- or less-pronounced motion triggers the sensor. Reducing the sensitivity level could require a moving object to be warmer or closer to the camera to trip it, for example.
CV offers more options for mitigating false alerts. One of the more popular is the ability to set motion detection zones. This feature lets you mask out parts of the camera’s field of view to tell the camera to ignore activity in those areas, or conversely to mask in specific areas to be monitored, effectively telling the camera to ignore activity everywhere else. It’s a fairly effective and user-friendly solution as it allows your camera to focus on locations where movement would most likely indicate a breach—windows and doors, for example—without getting confused by whatever other activity is going on in your home.
CV can also make quick judgement calls based on the geometric properties of a moving object. Something very small relative to the field of view is either a small object, like a floating dust mote, and deemed “uninteresting,” or it’s a larger object very far away and also not notable.
Increasingly, CV-based cameras are also including features like person detection and facial recognition as a way to cut down on false alerts. These go beyond the basic detection of a moving object and actually classify the object as a way to filter “important” from “unimportant” motion events.
There are a variety of CV algorithms that can power these features, and they’re often arranged in what Katsman calls a “funnel pattern,” where each step down the funnel increases the likelihood of positive detection. In a person detection example, the first step might be deciding the motion was significant enough. The next step would be deciding the moving object resembles a human form as opposed to an oblong, horizontal, or other-shaped object. The next step might recognize that the moving human shape has texture resembling skin or clothes. Finally, the algorithm recognizes the clothed moving human shape seems to have a face and decides it’s a human being. Alert sent.
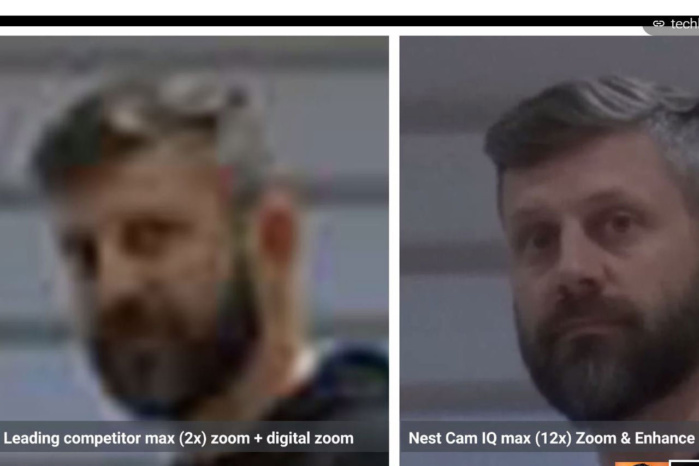
The Nest Cam IQ’s motion sensor works with its onboard 4K image sensor to identify faces.
Facial recognition is much more complex. It starts by trying to detect a face in the frame—usually this would be an extension of the person detection funnel, Katsman says. Then, depending on the desired accuracy, computational resources and design decisions, it will do one of two things. In one implementation it will attempt to identify facial features and their orientation in the 3D space, and then try to match those features to a database of predefined known faces. This method usually yields higher accuracy. In the other implementation, it tries to match the face to groups of images, each group representing a different person. The group closest to the face at hand would be considered the likely match.
Both of these methods require a pre-existing database of recognized individuals, which is why cameras with these features often require a few weeks of use before you start getting accurate results. The second method, however, can allow you to build such a database on the fly by letting you confirm or reject the identity of each face as it’s recognized—yes, that is Aunt Sally, no that’s not Uncle Joe—ostensibly speeding up the camera’s “learning” process.
The problem with these advanced features, as with CV detection as a whole, is their reliability is only as strong as the algorithms behind them. As we’ve seen in our testing, three different cameras with facial detection can yield wildly different results.
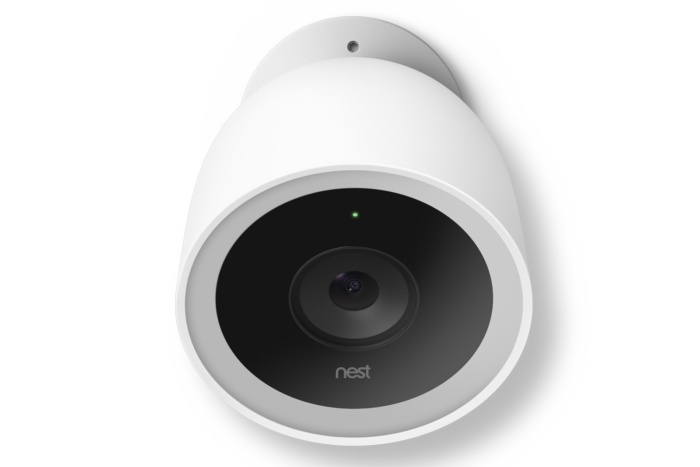
The Nest Cam IQ Outdoor lets you identify people and add them to a Familiar Faces library. It uses that information to distinguish between strangers and family and friends in its alerts.
“The concept of this approach is exciting, but in practice the results are often unreliable,” Bader says. “The more reliable solutions are very expensive, which is reflected in the camera’s price. The less complex solutions can be unreliable. This technology is still a work in process.”
Getting the most from your motion detection
While motion detection technology still has plenty of room for improvement, there is plenty you can do to increase your camera’s accuracy.
Start by heeding any placement instructions the manufacturer provides. Take advantage of any opportunity your camera gives you to help it learn by naming faces, tagging motion as interesting or uninteresting, and providing other feedback. This will help optimize the camera’s performance for your home environment. Experiment, too, with your camera’s motion sensitivity settings until you find the notification frequency that’s more accurate than annoying.
“At Canary, we find that many of our customers don’t change from the default settings,” Katsman says, “and they’re missing out on a great opportunity to help the device perform better for them.”
This story, “How your home security camera detects motion” was originally published by
TechHive.